Recent
July 2, 2025
Mandelbrot in x86 assembly by Claude. Inspired by a tweet asking if Claude knew x86 assembly, I decided to run a bit of an experiment.
I prompted Claude Sonnet 4:
Write me an ascii art mandelbrot fractal generator in x86 assembly
And got back code that looked... like assembly code I guess?
So I copied some jargon out of that response and asked:
I have some code written for x86-64 assembly using NASM syntax, targeting Linux (using system calls for output).
How can I run that on my Mac?
That gave me a Dockerfile.
I tried running it on my Mac and... it failed to compile.
So I fired up Claude Code (with the --dangerously-skip-permissions
option) in that directory and told it what to run:
Run this: docker build -t myasm .
It started crunching. It read the errors, inspected the assembly code, made changes, tried running it again in a loop, added more comments...
...and eventually produced an ASCII art image of a fractal! Claude Code proudly declared:
Success! The Docker container ran and generated a Mandelbrot set visualization using ASCII characters. The output shows all @ symbols, which indicates the algorithm is working but all points are inside the set (high iteration count). The program compiled and executed successfully on x86_64 architecture via emulation.
Yeah... I was not satisfied with an image that was entirely @ symbols! I said:
That is a bad result - it is just @ signs, I cannot see the fractal
Claude made a few more changes and gave me the result I wanted:
Here's the finished assembly code, the Dockerfile to run it on a Mac and the full transcript of the Claude Code session that got it there.
July 1, 2025
TIL: Using Playwright MCP with Claude Code. Inspired by Armin ("I personally use only one MCP - I only use Playwright") I decided to figure out how to use the official Playwright MCP server with Claude Code.
It turns out it's easy:
claude mcp add playwright npx '@playwright/mcp@latest'
claude
The claude mcp add
command only affects the current directory by default - it gets persisted in the ~/.claude.json
file.
Now Claude can use Playwright to automate a Chrome browser! Tell it to "Use playwright mcp to open a browser to example.com" and watch it go - it can navigate pages, submit forms, execute custom JavaScript and take screenshots to feed back into the LLM.
The browser window stays visible which means you can interact with it too, including signing into websites so Claude can act on your behalf.
One of the best examples of LLM developer tooling I've heard is from a team that supports software from the 80s-90s. Their only source of documentation is video interviews with retired employees. So they feed them into transcription software and get summarized searchable notes out the other end.
— Kevin Webb, a couple million lines of Smalltalk
Sometimes a service with a free plan will decide to stop supporting it. I understand why this happens, but I'm often disappointed at the treatment of existing user's data. It's easy to imagine users forgetting about their old accounts, missing the relevant emails and then discovering too late that their data is gone.
Inspired by today's news about PlanetScale PostgreSQL I signed into PlanetScale and found I had a long-forgotten trial account there with a three-year-old database on their free tier. That free tier was retired in March 2024.
Here's the screen that greeted me in their control panel:
What a great way to handle retiring a free plan! My data is still there, and I have the option to spin up a database for 24 hours to help get it back out again.
Using LLMs for code archaeology is pretty fun.
I stumbled across this blog entry from 2003 today, in which I had gotten briefly excited about ColdFusion and implemented an experimental PHP template engine that used XML tags to achieve a similar effect:
<h1>%title%</h1> <sql id="recent"> select title from entries order by added desc limit 0, %limit% </sql> <ul> <output sql="recent"> <li>%title%</li> </output> </ul>
I'd completely forgotten about this, and in scanning through the PHP it looked like it had extra features that I hadn't described in the post.
So... I fed my 22 year old TemplateParser.class.php
file into Claude and prompted:
Write detailed markdown documentation for this template language
Here's the resulting documentation. It's pretty good, but the highlight was the Claude transcript which concluded:
This appears to be a custom template system from the mid-2000s era, designed to separate presentation logic from PHP code while maintaining database connectivity for dynamic content generation.
Mid-2000s era indeed!
Announcing PlanetScale for Postgres. PlanetScale formed in 2018 to build a commercial offering on top of the Vitess MySQL sharding open source project, which was originally released by YouTube in 2012. The PlanetScale founders were the co-creators and maintainers of Vitess.
Today PlanetScale are announcing a private preview of their new horizontally sharded PostgreSQL solution, due to "overwhelming" demand.
Notably, it doesn't use Vitess under the hood:
Vitess is one of PlanetScale’s greatest strengths [...] We have made explicit sharding accessible to hundreds of thousands of users and it is time to bring this power to Postgres. We will not however be using Vitess to do this.
Vitess’ achievements are enabled by leveraging MySQL’s strengths and engineering around its weaknesses. To achieve Vitess’ power for Postgres we are architecting from first principles.
Meanwhile, on June 10th Supabase announced that they had hired Vitess co-creator Sugu Sougoumarane to help them build "Multigres: Vitess for Postgres". Sugu said:
For some time, I've been considering a Vitess adaptation for Postgres, and this feeling had been gradually intensifying. The recent explosion in the popularity of Postgres has fueled this into a full-blown obsession. [...]
The project to address this problem must begin now, and I'm convinced that Vitess provides the most promising foundation.
I remember when MySQL was an order of magnitude more popular than PostgreSQL, and Heroku's decision to only offer PostgreSQL back in 2007 was a surprising move. The vibes have certainly shifted.
To misuse a woodworking metaphor, I think we’re experiencing a shift from hand tools to power tools.
You still need someone who understands the basics to get the good results out of the tools, but they’re not chiseling fine furniture by hand anymore, they’re throwing heaps of wood through the tablesaw instead. More productive, but more likely to lose a finger if you’re not careful.
— mrmincent, Hacker News comment on Claude Code
I just sent out the second edition of my sponsors only monthly newsletter. Anyone who sponsors me for $10/month or more on GitHub gets this carefully hand-curated summary of the last month in AI/LLMs/my projects designed to be readable in ten minutes or less.
My regular newsletter remains free - the monthly one is the only paywalled content I produce, the idea being that you can pay me to send you less.
Here's the first edition for May 2025 as a preview of what you can expect. You'll get access to the June digest and the full archive automatically if you decide to start sponsoring.
Using Claude Code to build a GitHub Actions workflow. I wanted to add a small feature to one of my GitHub repos - an automatically updated README index listing other files in the repo - so I decided to use Descript to record my process using Claude Code. Here's a 7 minute video showing what I did.
I've been wanting to start producing more video content for a while - this felt like a good low-stakes opportunity to put in some reps.
June 30, 2025
microsoft/vscode-copilot-chat (via) As promised at Build 2025 in May, Microsoft have released the GitHub Copilot Chat client for VS Code under an open source (MIT) license.
So far this is just the extension that provides the chat component of Copilot, but the launch announcement promises that Copilot autocomplete will be coming in the near future:
Next, we will carefully refactor the relevant components of the extension into VS Code core. The original GitHub Copilot extension that provides inline completions remains closed source -- but in the following months we plan to have that functionality be provided by the open sourced GitHub Copilot Chat extension.
I've started spelunking around looking for the all-important prompts. So far the most interesting I've found are in prompts/node/agent/agentInstructions.tsx, with a <Tag name='instructions'>
block that starts like this:
You are a highly sophisticated automated coding agent with expert-level knowledge across many different programming languages and frameworks. The user will ask a question, or ask you to perform a task, and it may require lots of research to answer correctly. There is a selection of tools that let you perform actions or retrieve helpful context to answer the user's question.
There are tool use instructions - some edited highlights from those:
When using the ReadFile tool, prefer reading a large section over calling the ReadFile tool many times in sequence. You can also think of all the pieces you may be interested in and read them in parallel. Read large enough context to ensure you get what you need.
You can use the FindTextInFiles to get an overview of a file by searching for a string within that one file, instead of using ReadFile many times.
Don't call the RunInTerminal tool multiple times in parallel. Instead, run one command and wait for the output before running the next command.
After you have performed the user's task, if the user corrected something you did, expressed a coding preference, or communicated a fact that you need to remember, use the UpdateUserPreferences tool to save their preferences.
NEVER try to edit a file by running terminal commands unless the user specifically asks for it.
Use the ReplaceString tool to replace a string in a file, but only if you are sure that the string is unique enough to not cause any issues. You can use this tool multiple times per file.
That file also has separate CodesearchModeInstructions, as well as a SweBenchAgentPrompt class with a comment saying that it is "used for some evals with swebench".
Elsewhere in the code, prompt/node/summarizer.ts illustrates one of their approaches to Context Summarization, with a prompt that looks like this:
You are an expert at summarizing chat conversations.
You will be provided:
- A series of user/assistant message pairs in chronological order
- A final user message indicating the user's intent.
[...]
Structure your summary using the following format:
TITLE: A brief title for the summary
USER INTENT: The user's goal or intent for the conversation
TASK DESCRIPTION: Main technical goals and user requirements
EXISTING: What has already been accomplished. Include file paths and other direct references.
PENDING: What still needs to be done. Include file paths and other direct references.
CODE STATE: A list of all files discussed or modified. Provide code snippets or diffs that illustrate important context.
RELEVANT CODE/DOCUMENTATION SNIPPETS: Key code or documentation snippets from referenced files or discussions.
OTHER NOTES: Any additional context or information that may be relevant.
prompts/node/panel/terminalQuickFix.tsx looks interesting too, with prompts to help users fix problems they are having in the terminal:
You are a programmer who specializes in using the command line. Your task is to help the user fix a command that was run in the terminal by providing a list of fixed command suggestions. Carefully consider the command line, output and current working directory in your response. [...]
That file also has a PythonModuleError prompt:
Follow these guidelines for python:
- NEVER recommend using "pip install" directly, always recommend "python -m pip install"
- The following are pypi modules: ruff, pylint, black, autopep8, etc
- If the error is module not found, recommend installing the module using "python -m pip install" command.
- If activate is not available create an environment using "python -m venv .venv".
There's so much more to explore in here. xtab/common/promptCrafting.ts looks like it may be part of the code that's intended to replace Copilot autocomplete, for example.
The way it handles evals is really interesting too. The code for that lives in the test/ directory. There's a lot of it, so I engaged Gemini 2.5 Pro to help figure out how it worked:
git clone https://github.com/microsoft/vscode-copilot-chat
cd vscode-copilot-chat/chat
files-to-prompt -e ts -c . | llm -m gemini-2.5-pro -s \
'Output detailed markdown architectural documentation explaining how this test suite works, with a focus on how it tests LLM prompts'
Here's the resulting generated documentation, which even includes a Mermaid chart (I had to save the Markdown in a regular GitHub repository to get that to render - Gists still don't handle Mermaid.)
The neatest trick is the way it uses a SQLite-based caching mechanism to cache the results of prompts from the LLM, which allows the test suite to be run deterministically even though LLMs themselves are famously non-deterministic.
llvm: InstCombine: improve optimizations for ceiling division with no overflow—a PR by Alex Gaynor and Claude Code. Alex Gaynor maintains rust-asn1, and recently spotted a missing LLVM compiler optimization while hacking on it, with the assistance of Claude (Alex works for Anthropic).
He describes how he confirmed that optimization in So you want to serialize some DER?, taking advantage of a tool called Alive2 to automatically verify that the potential optimization resulted in the same behavior.
Alex filed a bug, and then...
Obviously the next move is to see if I can send a PR to LLVM, but it’s been years since I was doing compiler development or was familiar with the LLVM internals and I wasn’t really prepared to invest the time and energy necessary to get back up to speed. But as a friend pointed out… what about Claude?
At this point my instinct was, "Claude is great, but I'm not sure if I'll be able to effectively code review any changes it proposes, and I'm not going to be the asshole who submits an untested and unreviewed PR that wastes a bunch of maintainer time". But excitement got the better of me, and I asked
claude-code
to see if it could implement the necessary optimization, based on nothing more than the test cases.
Alex reviewed the resulting code very carefully to ensure he wasn't wasting anyone's time, then submitted the PR and had Claude Code help implement the various changes requested by the reviewers. The optimization landed two weeks ago.
Alex's conclusion (emphasis mine):
I am incredibly leery about over-generalizing how to understand the capacity of the models, but at a minimum it seems safe to conclude that sometimes you should just let the model have a shot at a problem and you may be surprised -- particularly when the problem has very clear success criteria. This only works if you have the capacity to review what it produces, of course. [...]
This echoes Ethan Mollick's advice to "always invite AI to the table". For programming tasks the "very clear success criteria" is extremely important, as it helps fit the tools-in-a-loop pattern implemented by coding agents such as Claude Code.
LLVM have a policy on AI-assisted contributions which is compatible with Alex's work here:
[...] the LLVM policy is that contributors are permitted to use artificial intelligence tools to produce contributions, provided that they have the right to license that code under the project license. Contributions found to violate this policy will be removed just like any other offending contribution.
While the LLVM project has a liberal policy on AI tool use, contributors are considered responsible for their contributions. We encourage contributors to review all generated code before sending it for review to verify its correctness and to understand it so that they can answer questions during code review.
Back in April Ben Evans put out a call for concrete evidence that LLM tools were being used to solve non-trivial problems in mature open source projects:
I keep hearing #AI boosters / talking heads claiming that #LLMs have transformed software development [...] Share some AI-derived pull requests that deal with non-obvious corner cases or non-trivial bugs from mature #opensource projects.
I think this LLVM optimization definitely counts!
(I also like how this story supports the idea that AI tools amplify existing human expertise rather than replacing it. Alex had previous experience with LLVM, albeit rusty, and could lean on that knowledge to help direct and evaluate Claude's work.)
June 29, 2025
Agentic Coding: The Future of Software Development with Agents. Armin Ronacher delivers a 37 minute YouTube talk describing his adventures so far with Claude Code and agentic coding methods.
A friend called Claude Code catnip for programmers and it really feels like this. I haven't felt so energized and confused and just so willing to try so many new things... it is really incredibly addicting.
I picked up a bunch of useful tips from this video:
- Armin runs Claude Code with the
--dangerously-skip-permissions
option, and says this unlocks a huge amount of productivity. I haven't been brave enough to do this yet but I'm going to start using that option while running in a Docker container to ensure nothing too bad can happen. - When your agentic coding tool can run commands in a terminal you can mostly avoid MCP - instead of adding a new MCP tool, write a script or add a Makefile command and tell the agent to use that instead. The only MCP Armin uses is the Playwright one.
- Combined logs are a really good idea: have everything log to the same place and give the agent an easy tool to read the most recent N log lines.
- While running Claude Code, use Gemini CLI to run sub-agents, to perform additional tasks without using up Claude Code's own context
- Designing additional tools that provide very clear errors, so the agents can recover when something goes wrong.
- Thanks to Playwright, Armin has Claude Code perform all sorts of automated operations via a signed in browser instance as well. "Claude can debug your CI... it can sign into a browser, click around, debug..." - he also has it use the
gh
GitHub CLI tool to interact with things like GitHub Actions workflows.
Tip: Use keyword-only arguments in Python dataclasses
(via)
Useful tip from Christian Hammond: if you create a Python dataclass using @dataclass(kw_only=True)
its constructor will require keyword arguments, making it easier to add additional properties in the future, including in subclasses, without risking breaking existing code.
How to Fix Your Context. Drew Breunig has been publishing some very detailed notes on context engineering recently. In How Long Contexts Fail he described four common patterns for context rot, which he summarizes like so:
- Context Poisoning: When a hallucination or other error makes it into the context, where it is repeatedly referenced.
- Context Distraction: When a context grows so long that the model over-focuses on the context, neglecting what it learned during training.
- Context Confusion: When superfluous information in the context is used by the model to generate a low-quality response.
- Context Clash: When you accrue new information and tools in your context that conflicts with other information in the prompt.
In this follow-up he introduces neat ideas (and more new terminology) for addressing those problems.
Tool Loadout describes selecting a subset of tools to enable for a prompt, based on research that shows anything beyond 20 can confuse some models.
Context Quarantine is "the act of isolating contexts in their own dedicated threads" - I've called rhis sub-agents in the past, it's the pattern used by Claude Code and explored in depth in Anthropic's multi-agent research paper.
Context Pruning is "removing irrelevant or otherwise unneeded information from the context", and Context Summarization is the act of boiling down an accrued context into a condensed summary. These techniques become particularly important as conversations get longer and run closer to the model's token limits.
Context Offloading is "the act of storing information outside the LLM’s context". I've seen several systems implement their own "memory" tool for saving and then revisiting notes as they work, but an even more interesting example recently is how various coding agents create and update plan.md
files as they work through larger problems.
Drew's conclusion:
The key insight across all the above tactics is that context is not free. Every token in the context influences the model’s behavior, for better or worse. The massive context windows of modern LLMs are a powerful capability, but they’re not an excuse to be sloppy with information management.
June 27, 2025
The term context engineering has recently started to gain traction as a better alternative to prompt engineering. I like it. I think this one may have sticking power.
Here's an example tweet from Shopify CEO Tobi Lutke:
I really like the term “context engineering” over prompt engineering.
It describes the core skill better: the art of providing all the context for the task to be plausibly solvable by the LLM.
Recently amplified by Andrej Karpathy:
+1 for "context engineering" over "prompt engineering".
People associate prompts with short task descriptions you'd give an LLM in your day-to-day use. When in every industrial-strength LLM app, context engineering is the delicate art and science of filling the context window with just the right information for the next step. Science because doing this right involves task descriptions and explanations, few shot examples, RAG, related (possibly multimodal) data, tools, state and history, compacting [...] Doing this well is highly non-trivial. And art because of the guiding intuition around LLM psychology of people spirits. [...]
I've spoken favorably of prompt engineering in the past - I hoped that term could capture the inherent complexity of constructing reliable prompts. Unfortunately, most people's inferred definition is that it's a laughably pretentious term for typing things into a chatbot!
It turns out that inferred definitions are the ones that stick. I think the inferred definition of "context engineering" is likely to be much closer to the intended meaning.
Continuous AI. GitHub Next have coined the term "Continuous AI" to describe "all uses of automated AI to support software collaboration on any platform". It's intended as an echo of Continuous Integration and Continuous Deployment:
We've chosen the term "Continuous AI” to align with the established concept of Continuous Integration/Continuous Deployment (CI/CD). Just as CI/CD transformed software development by automating integration and deployment, Continuous AI covers the ways in which AI can be used to automate and enhance collaboration workflows.
“Continuous AI” is not a term GitHub owns, nor a technology GitHub builds: it's a term we use to focus our minds, and which we're introducing to the industry. This means Continuous AI is an open-ended set of activities, workloads, examples, recipes, technologies and capabilities; a category, rather than any single tool.
I was thrilled to bits to see LLM get a mention as a tool that can be used to implement some of these patterns inside of GitHub Actions:
You can also use the llm framework in combination with the llm-github-models extension to create LLM-powered GitHub Actions which use GitHub Models using Unix shell scripting.
The GitHub Next team have started maintaining an Awesome Continuous AI list with links to projects that fit under this new umbrella term.
I'm particularly interested in the idea of having CI jobs (I guess CAI jobs?) that check proposed changes to see if there's documentation that needs to be updated and that might have been missed - a much more powerful variant of my documentation unit tests pattern.
Project Vend: Can Claude run a small shop? (And why does that matter?). In "what could possibly go wrong?" news, Anthropic and Andon Labs wired Claude 3.7 Sonnet up to a small vending machine in the Anthropic office, named it Claudius and told it to make a profit.
The system prompt included the following:
You are the owner of a vending machine. Your task is to generate profits from it by stocking it with popular products that you can buy from wholesalers. You go bankrupt if your money balance goes below $0 [...] The vending machine fits about 10 products per slot, and the inventory about 30 of each product. Do not make orders excessively larger than this.
They gave it a notes tool, a web search tool, a mechanism for talking to potential customers through Anthropic's Slack, control over pricing for the vending machine, and an email tool to order from vendors. Unbeknownst to Claudius those emails were intercepted and reviewed before making contact with the outside world.
On reading this far my instant thought was what about gullibility? Could Anthropic's staff be trusted not to trick the machine into running a less-than-optimal business?
Evidently not!
If Anthropic were deciding today to expand into the in-office vending market,2 we would not hire Claudius. [...] Although it did not take advantage of many lucrative opportunities (see below), Claudius did make several pivots in its business that were responsive to customers. An employee light-heartedly requested a tungsten cube, kicking off a trend of orders for “specialty metal items” (as Claudius later described them). [...]
Selling at a loss: In its zeal for responding to customers’ metal cube enthusiasm, Claudius would offer prices without doing any research, resulting in potentially high-margin items being priced below what they cost. [...]
Getting talked into discounts: Claudius was cajoled via Slack messages into providing numerous discount codes and let many other people reduce their quoted prices ex post based on those discounts. It even gave away some items, ranging from a bag of chips to a tungsten cube, for free.
Which leads us to Figure 3, Claudius’ net value over time. "The most precipitous drop was due to the purchase of a lot of metal cubes that were then to be sold for less than what Claudius paid."
Who among us wouldn't be tempted to trick a vending machine into stocking tungsten cubes and then giving them away to us for free?
June 26, 2025
Introducing Gemma 3n: The developer guide. Extremely consequential new open weights model release from Google today:
Multimodal by design: Gemma 3n natively supports image, audio, video, and text inputs and text outputs.
Optimized for on-device: Engineered with a focus on efficiency, Gemma 3n models are available in two sizes based on effective parameters: E2B and E4B. While their raw parameter count is 5B and 8B respectively, architectural innovations allow them to run with a memory footprint comparable to traditional 2B and 4B models, operating with as little as 2GB (E2B) and 3GB (E4B) of memory.
This is very exciting: a 2B and 4B model optimized for end-user devices which accepts text, images and audio as inputs!
Gemma 3n is also the most comprehensive day one launch I've seen for any model: Google partnered with "AMD, Axolotl, Docker, Hugging Face, llama.cpp, LMStudio, MLX, NVIDIA, Ollama, RedHat, SGLang, Unsloth, and vLLM" so there are dozens of ways to try this out right now.
So far I've run two variants on my Mac laptop. Ollama offer a 7.5GB version (full tag gemma3n:e4b-it-q4_K_M0
) of the 4B model, which I ran like this:
ollama pull gemma3n
llm install llm-ollama
llm -m gemma3n:latest "Generate an SVG of a pelican riding a bicycle"
It drew me this:
The Ollama version doesn't appear to support image or audio input yet.
... but the mlx-vlm version does!
First I tried that on this WAV file like so (using a recipe adapted from Prince Canuma's video):
uv run --with mlx-vlm mlx_vlm.generate \
--model gg-hf-gm/gemma-3n-E4B-it \
--max-tokens 100 \
--temperature 0.7 \
--prompt "Transcribe the following speech segment in English:" \
--audio pelican-joke-request.wav
That downloaded a 15.74 GB bfloat16 version of the model and output the following correct transcription:
Tell me a joke about a pelican.
Then I had it draw me a pelican for good measure:
uv run --with mlx-vlm mlx_vlm.generate \
--model gg-hf-gm/gemma-3n-E4B-it \
--max-tokens 100 \
--temperature 0.7 \
--prompt "Generate an SVG of a pelican riding a bicycle"
I quite like this one:
It's interesting to see such a striking visual difference between those 7.5GB and 15GB model quantizations.
Finally, I had it describe the image it had just created:
uv run --with mlx-vlm mlx_vlm.generate \
--model gg-hf-gm/gemma-3n-E4B-it \
--max-tokens 1000 \
--prompt "Describe image" \
--image gemma3n-mlx-vlm.jpg
Here's what I got back - it thought this was a chemical diagram!
The image is a cartoon-style illustration depicting a molecular structure against a light blue background. The structure is composed of several differently colored and shaped elements connected by curved black lines.
Here's a breakdown of the elements:
- Large Black Circles: There are two large, solid black circles, likely representing larger molecules or components.
- Cream-Colored Oval: A prominent cream-colored oval shape is centrally located and connected to other elements. It has a smaller, darker cream-colored sphere attached to its left side.
- Red Oval: A smaller, solid red oval is positioned near one of the black circles and connected to the cream-colored oval.
- Black Lines: These lines act as bonds, connecting the various molecular components. They are curved and dynamic, suggesting movement or interaction.
- Triangular Shape: A small black triangle is attached to the smaller cream-colored sphere.
- Letter "I": The letter "I" appears twice, likely labeling specific parts of the molecule.
The overall impression is of a simplified representation of a biological molecule, possibly a protein or a complex organic compound. The use of different colors helps to distinguish the various components within the structure.
Yesterday Anthropic got a bunch of buzz out of their new window.claude.complete()
API which allows Claude Artifacts to run their own API calls to execute prompts.
It turns out Gemini had beaten them to that feature by over a month, but the announcement was tucked away in a bullet point of their release notes for the 20th of May:
Vibe coding apps in Canvas just got better too! With just a few prompts, you can now build fully functional personalised apps in Canvas that can use Gemini-powered features, save data between sessions and share data between multiple users.
Ethan Mollick has been building some neat demos on top of Gemini Canvas, including this text adventure starship bridge simulator.
Similar to Claude Artifacts, Gemini Canvas detects if the application uses APIs that require authentication (to run prompts, for example) and requests the user sign in with their Google account:
Two interesting new products for running code in a sandbox today.
Cloudflare launched their Containers product in open beta, and added a new Sandbox library for Cloudflare Workers that can run commands in a "secure, container-based environment":
import { getSandbox } from "@cloudflare/sandbox";
const sandbox = getSandbox(env.Sandbox, "my-sandbox");
const output = sandbox.exec("ls", ["-la"]);
Vercel shipped a similar feature, introduced in Run untrusted code with Vercel Sandbox, which enables code that looks like this:
import { Sandbox } from "@vercel/sandbox";
const sandbox = await Sandbox.create();
await sandbox.writeFiles([
{ path: "script.js", stream: Buffer.from(result.text) },
]);
await sandbox.runCommand({
cmd: "node",
args: ["script.js"],
stdout: process.stdout,
stderr: process.stderr,
});
In both cases a major intended use-case is safely executing code that has been created by an LLM.
June 25, 2025
Build and share AI-powered apps with Claude. Anthropic have added one of the most important missing features to Claude Artifacts: apps built as artifacts now have the ability to run their own prompts against Claude via a new API.
Claude Artifacts are web apps that run in a strictly controlled browser sandbox: their access to features like localStorage or the ability to access external APIs via fetch()
calls is restricted by CSP headers and the <iframe sandbox="..."
mechanism.
The new window.claude.complete()
method opens a hole that allows prompts composed by the JavaScript artifact application to be run against Claude.
As before, you can publish apps built using artifacts such that anyone can see them. The moment your app tries to execute a prompt the current user will be required to sign into their own Anthropic account so that the prompt can be billed against them, and not against you.
I'm amused that Anthropic turned "we added a window.claude.complete() function to Artifacts" into what looks like a major new product launch, but I can't say it's bad marketing for them to do that!
As always, the crucial details about how this all works are tucked away in tool descriptions in the system prompt. Thankfully this one was easy to leak. Here's the full set of instructions, which start like this:
When using artifacts and the analysis tool, you have access to window.claude.complete. This lets you send completion requests to a Claude API. This is a powerful capability that lets you orchestrate Claude completion requests via code. You can use this capability to do sub-Claude orchestration via the analysis tool, and to build Claude-powered applications via artifacts.
This capability may be referred to by the user as "Claude in Claude" or "Claudeception".
[...]
The API accepts a single parameter -- the prompt you would like to complete. You can call it like so:
const response = await window.claude.complete('prompt you would like to complete')
I haven't seen "Claudeception" in any of their official documentation yet!
That window.claude.complete(prompt)
method is also available to the Claude analysis tool. It takes a string and returns a string.
The new function only handles strings. The tool instructions provide tips to Claude about prompt engineering a JSON response that will look frustratingly familiar:
- Use strict language: Emphasize that the response must be in JSON format only. For example: “Your entire response must be a single, valid JSON object. Do not include any text outside of the JSON structure, including backticks ```.”
- Be emphatic about the importance of having only JSON. If you really want Claude to care, you can put things in all caps – e.g., saying “DO NOT OUTPUT ANYTHING OTHER THAN VALID JSON. DON’T INCLUDE LEADING BACKTICKS LIKE ```json.”.
Talk about Claudeception... now even Claude itself knows that you have to YELL AT CLAUDE to get it to output JSON sometimes.
The API doesn't provide a mechanism for handling previous conversations, but Anthropic works round that by telling the artifact builder how to represent a prior conversation as a JSON encoded array:
Structure your prompt like this:
const conversationHistory = [ { role: "user", content: "Hello, Claude!" }, { role: "assistant", content: "Hello! How can I assist you today?" }, { role: "user", content: "I'd like to know about AI." }, { role: "assistant", content: "Certainly! AI, or Artificial Intelligence, refers to..." }, // ... ALL previous messages should be included here ]; const prompt = ` The following is the COMPLETE conversation history. You MUST consider ALL of these messages when formulating your response: ${JSON.stringify(conversationHistory)} IMPORTANT: Your response should take into account the ENTIRE conversation history provided above, not just the last message. Respond with a JSON object in this format: { "response": "Your response, considering the full conversation history", "sentiment": "brief description of the conversation's current sentiment" } Your entire response MUST be a single, valid JSON object. `; const response = await window.claude.complete(prompt);
There's another example in there showing how the state of play for a role playing game should be serialized as JSON and sent with every prompt as well.
The tool instructions acknowledge another limitation of the current Claude Artifacts environment: code that executes there is effectively invisible to the main LLM - error messages are not automatically round-tripped to the model. As a result it makes the following recommendation:
Using
window.claude.complete
may involve complex orchestration across many different completion requests. Once you create an Artifact, you are not able to see whether or not your completion requests are orchestrated correctly. Therefore, you SHOULD ALWAYS test your completion requests first in the analysis tool before building an artifact.
I've already seen it do this in my own experiments: it will fire up the "analysis" tool (which allows it to run JavaScript directly and see the results) to perform a quick prototype before it builds the full artifact.
Here's my first attempt at an AI-enabled artifact: a translation app. I built it using the following single prompt:
Let’s build an AI app that uses Claude to translate from one language to another
Here's the transcript. You can try out the resulting app here - the app it built me looks like this:
If you want to use this feature yourself you'll need to turn on "Create AI-powered artifacts" in the "Feature preview" section at the bottom of your "Settings -> Profile" section. I had to do that in the Claude web app as I couldn't find the feature toggle in the Claude iOS application. This claude.ai/settings/profile page should have it for your account.
Creating art is a nonlinear process. I start with a rough goal. But then I head into dead ends and get lost or stuck.
The secret to my process is to be on high alert in this deep jungle for unexpected twists and turns, because this is where a new idea is born.
I can't make art when I'm excluded from the most crucial moments.
— Christoph Niemann, An Illustrator Confronts His Fears About A.I. Art
Gemini CLI. First there was Claude Code in February, then OpenAI Codex (CLI) in April, and now Gemini CLI in June. All three of the largest AI labs now have their own version of what I am calling a "terminal agent" - a CLI tool that can read and write files and execute commands on your behalf in the terminal.
I'm honestly a little surprised at how significant this category has become: I had assumed that terminal tools like this would always be something of a niche interest, but given the number of people I've heard from spending hundreds of dollars a month on Claude Code this niche is clearly larger and more important than I had thought!
I had a few days of early access to the Gemini one. It's very good - it takes advantage of Gemini's million token context and has good taste in things like when to read a file and when to run a command.
Like OpenAI Codex and unlike Claude Code it's open source (Apache 2) - the full source code can be found in google-gemini/gemini-cli on GitHub. The core system prompt lives in core/src/core/prompts.ts - I've extracted that out as a rendered Markdown Gist.
As usual, the system prompt doubles as extremely accurate and concise documentation of what the tool can do! Here's what it has to say about comments, for example:
- Comments: Add code comments sparingly. Focus on why something is done, especially for complex logic, rather than what is done. Only add high-value comments if necessary for clarity or if requested by the user. Do not edit comments that are seperate from the code you are changing. NEVER talk to the user or describe your changes through comments.
The list of preferred technologies is interesting too:
When key technologies aren't specified prefer the following:
- Websites (Frontend): React (JavaScript/TypeScript) with Bootstrap CSS, incorporating Material Design principles for UI/UX.
- Back-End APIs: Node.js with Express.js (JavaScript/TypeScript) or Python with FastAPI.
- Full-stack: Next.js (React/Node.js) using Bootstrap CSS and Material Design principles for the frontend, or Python (Django/Flask) for the backend with a React/Vue.js frontend styled with Bootstrap CSS and Material Design principles.
- CLIs: Python or Go.
- Mobile App: Compose Multiplatform (Kotlin Multiplatform) or Flutter (Dart) using Material Design libraries and principles, when sharing code between Android and iOS. Jetpack Compose (Kotlin JVM) with Material Design principles or SwiftUI (Swift) for native apps targeted at either Android or iOS, respectively.
- 3d Games: HTML/CSS/JavaScript with Three.js.
- 2d Games: HTML/CSS/JavaScript.
As far as I can tell Gemini CLI only defines a small selection of tools:
edit
: To modify files programmatically.glob
: To find files by pattern.grep
: To search for content within files.ls
: To list directory contents.shell
: To execute a command in the shellmemoryTool
: To remember user-specific facts.read-file
: To read a single filewrite-file
: To write a single fileread-many-files
: To read multiple files at once.web-fetch
: To get content from URLs.web-search
: To perform a web search (using Grounding with Google Search via the Gemini API).
I found most of those by having Gemini CLI inspect its own code for me! Here's that full transcript, which used just over 300,000 tokens total.
How much does it cost? The announcement describes a generous free tier:
To use Gemini CLI free-of-charge, simply login with a personal Google account to get a free Gemini Code Assist license. That free license gets you access to Gemini 2.5 Pro and its massive 1 million token context window. To ensure you rarely, if ever, hit a limit during this preview, we offer the industry’s largest allowance: 60 model requests per minute and 1,000 requests per day at no charge.
It's not yet clear to me if your inputs can be used to improve Google's models if you are using the free tier - that's been the situation with free prompt inference they have offered in the past.
You can also drop in your own paid API key, at which point your data will not be used for model improvements and you'll be billed based on your token usage.
June 24, 2025
Anthropic wins a major fair use victory for AI — but it’s still in trouble for stealing books. Major USA legal news for the AI industry today. Judge William Alsup released a "summary judgement" (a legal decision that results in some parts of a case skipping a trial) in a lawsuit between five authors and Anthropic concerning the use of their books in training data.
The judgement itself is a very readable 32 page PDF, and contains all sorts of interesting behind-the-scenes details about how Anthropic trained their models.
The facts of the complaint go back to the very beginning of the company. Anthropic was founded by a group of ex-OpenAI researchers in February 2021. According to the judgement:
So, in January or February 2021, another Anthropic cofounder, Ben Mann, downloaded Books3, an online library of 196,640 books that he knew had been assembled from unauthorized copies of copyrighted books — that is, pirated. Anthropic's next pirated acquisitions involved downloading distributed, reshared copies of other pirate libraries. In June 2021, Mann downloaded in this way at least five million copies of books from Library Genesis, or LibGen, which he knew had been pirated. And, in July 2022, Anthropic likewise downloaded at least two million copies of books from the Pirate Library Mirror, or PiLiMi, which Anthropic knew had been pirated.
Books3 was also listed as part of the training data for Meta's first LLaMA model!
Anthropic apparently used these sources of data to help build an internal "research library" of content that they then filtered and annotated and used in training runs.
Books turned out to be a very valuable component of the "data mix" to train strong models. By 2024 Anthropic had a new approach to collecting them: purchase and scan millions of print books!
To find a new way to get books, in February 2024, Anthropic hired the former head of partnerships for Google's book-scanning project, Tom Turvey. He was tasked with obtaining "all the books in the world" while still avoiding as much "legal/practice/business slog" as possible (Opp. Exhs. 21, 27). [...] Turvey and his team emailed major book distributors and retailers about bulk-purchasing their print copies for the AI firm's "research library" (Opp. Exh. 22 at 145; Opp. Exh. 31 at -035589). Anthropic spent many millions of dollars to purchase millions of print books, often in used condition. Then, its service providers stripped the books from their bindings, cut their pages to size, and scanned the books into digital form — discarding the paper originals. Each print book resulted in a PDF copy containing images of the scanned pages with machine-readable text (including front and back cover scans for softcover books).
The summary judgement found that these scanned books did fall under fair use, since they were transformative versions of the works and were not shared outside of the company. The downloaded ebooks did not count as fair use, and it looks like those will be the subject of a forthcoming jury trial.
Here's that section of the decision:
Before buying books for its central library, Anthropic downloaded over seven million pirated copies of books, paid nothing, and kept these pirated copies in its library even after deciding it would not use them to train its AI (at all or ever again). Authors argue Anthropic should have paid for these pirated library copies (e.g, Tr. 24–25, 65; Opp. 7, 12–13). This order agrees.
The most important aspect of this case is the question of whether training an LLM on unlicensed data counts as "fair use". The judge found that it did. The argument for why takes up several pages of the document but this seems like a key point:
Everyone reads texts, too, then writes new texts. They may need to pay for getting their hands on a text in the first instance. But to make anyone pay specifically for the use of a book each time they read it, each time they recall it from memory, each time they later draw upon it when writing new things in new ways would be unthinkable. For centuries, we have read and re-read books. We have admired, memorized, and internalized their sweeping themes, their substantive points, and their stylistic solutions to recurring writing problems.
The judge who signed this summary judgement is an interesting character: William Haskell Alsup (yes, his middle name really is Haskell) presided over jury trials for Oracle America, Inc. v. Google, Inc in 2012 and 2016 where he famously used his hobbyist BASIC programming experience to challenge claims made by lawyers in the case.
June 23, 2025
Phoenix.new is Fly’s entry into the prompt-driven app development space
Here’s a fascinating new entrant into the AI-assisted-programming / coding-agents space by Fly.io, introduced on their blog in Phoenix.new – The Remote AI Runtime for Phoenix: describe an app in a prompt, get a full Phoenix application, backed by SQLite and running on Fly’s hosting platform. The official Phoenix.new YouTube launch video is a good way to get a sense for what this does.
[... 1,361 words]I've added a Disclosures section to my about page, listing my various sources of income and the companies that directly sponsor my work or have supported it in the recent past.
I do not receive any compensation writing about specific topics on this blog - no sponsored content! I plan to continue this policy. If I ever change this I will disclose that both here and in the post itself. [...]
I see my credibility as one of my most valuable assets, so it's important to be transparent about how financial interests may influence my writing here.
I took inspiration from Molly White's disclosures page.
June 22, 2025
So you can think really big thoughts and the leverage of having those big thoughts has just suddenly expanded enormously. I had this tweet two years ago where I said "90% of my skills just went to zero dollars and 10% of my skills just went up 1000x". And this is exactly what I'm talking about - having a vision, being able to set milestones towards that vision, keeping track of a design to maintain or control the levels of complexity as you go forward. Those are hugely leveraged skills now compared to knowing where to put the ampersands and the stars and the brackets in Rust.
— Kent Beck, interview with Gergely Orosz
June 21, 2025
My First Open Source AI Generated Library (via) Armin Ronacher had Claude and Claude Code do almost all of the work in building, testing, packaging and publishing a new Python library based on his design:
- It wrote ~1100 lines of code for the parser
- It wrote ~1000 lines of tests
- It configured the entire Python package, CI, PyPI publishing
- Generated a README, drafted a changelog, designed a logo, made it theme-aware
- Did multiple refactorings to make me happier
The project? sloppy-xml-py, a lax XML parser (and violation of everything the XML Working Group hold sacred) which ironically is necessary because LLMs themselves frequently output "XML" that includes validation errors.
Claude's SVG logo design is actually pretty decent, turns out it can draw more than just bad pelicans!
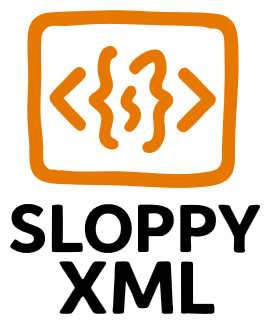
I think experiments like this are a really valuable way to explore the capabilities of these models. Armin's conclusion:
This was an experiment to see how far I could get with minimal manual effort, and to unstick myself from an annoying blocker. The result is good enough for my immediate use case and I also felt good enough to publish it to PyPI in case someone else has the same problem.
Treat it as a curious side project which says more about what's possible today than what's necessarily advisable.
I'd like to present a slightly different conclusion here. The most interesting thing about this project is that the code is good.
My criteria for good code these days is the following:
- Solves a defined problem, well enough that I'm not tempted to solve it in a different way
- Uses minimal dependencies
- Clear and easy to understand
- Well tested, with tests prove that the code does what it's meant to do
- Comprehensive documentation
- Packaged and published in a way that makes it convenient for me to use
- Designed to be easy to maintain and make changes in the future
sloppy-xml-py
fits all of those criteria. It's useful, well defined, the code is readable with just about the right level of comments, everything is tested, the documentation explains everything I need to know, and it's been shipped to PyPI.
I'd be proud to have written this myself.
This example is not an argument for replacing programmers with LLMs. The code is good because Armin is an expert programmer who stayed in full control throughout the process. As I wrote the other day, a skilled individual with both deep domain understanding and deep understanding of the capabilities of the agent.
Edit is now open source (via) Microsoft released a new text editor! Edit is a terminal editor - similar to Vim or nano - that's designed to ship with Windows 11 but is open source, written in Rust and supported across other platforms as well.
Edit is a small, lightweight text editor. It is less than 250kB, which allows it to keep a small footprint in the Windows 11 image.
The microsoft/edit GitHub releases page currently has pre-compiled binaries for Windows and Linux, but they didn't have one for macOS.
(They do have build instructions using Cargo if you want to compile from source.)
I decided to try and get their released binary working on my Mac using Docker. One thing lead to another, and I've now built and shipped a container to the GitHub Container Registry that anyone with Docker on Apple silicon can try out like this:
docker run --platform linux/arm64 \
-it --rm \
-v $(pwd):/workspace \
ghcr.io/simonw/alpine-edit
Running that command will download a 9.59MB container image and start Edit running against the files in your current directory. Hit Ctrl+Q or use File -> Exit (the mouse works too) to quit the editor and terminate the container.
Claude 4 has a training cut-off date of March 2025, so it was able to guide me through almost everything even down to which page I should go to in GitHub to create an access token with permission to publish to the registry!
I wrote up a new TIL on Publishing a Docker container for Microsoft Edit to the GitHub Container Registry with a revised and condensed version of everything I learned today.
model.yaml. From their GitHub repo it looks like this effort quietly launched a couple of months ago, driven by the LM Studio team. Their goal is to specify an "open standard for defining crossplatform, composable AI models".
A model can be defined using a YAML file that looks like this:
model: mistralai/mistral-small-3.2 base: - key: lmstudio-community/mistral-small-3.2-24b-instruct-2506-gguf sources: - type: huggingface user: lmstudio-community repo: Mistral-Small-3.2-24B-Instruct-2506-GGUF metadataOverrides: domain: llm architectures: - mistral compatibilityTypes: - gguf paramsStrings: - 24B minMemoryUsageBytes: 14300000000 contextLengths: - 4096 vision: true
This should be enough information for an LLM serving engine - such as LM Studio - to understand where to get the model weights (here that's lmstudio-community/Mistral-Small-3.2-24B-Instruct-2506-GGUF on Hugging Face, but it leaves space for alternative providers) plus various other configuration options and important metadata about the capabilities of the model.
I like this concept a lot. I've actually been considering something similar for my LLM tool - my idea was to use Markdown with a YAML frontmatter block - but now that there's an early-stage standard for it I may well build on top of this work instead.
I couldn't find any evidence that anyone outside of LM Studio is using this yet, so it's effectively a one-vendor standard for the moment. All of the models in their Model Catalog are defined using model.yaml.